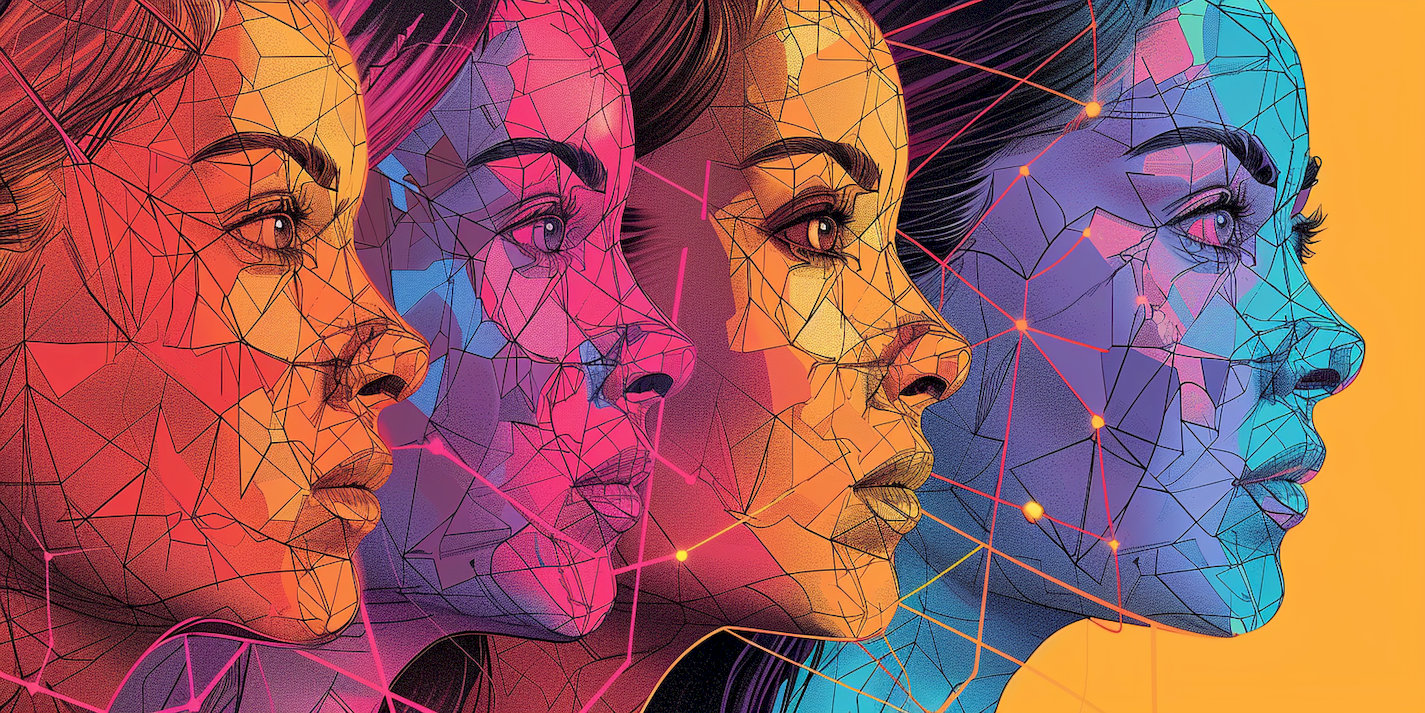
Artificial intelligence (AI) is rapidly transforming various aspects of our lives, from healthcare and education to finance and transportation. While AI holds immense potential to enhance efficiency, improve decision-making, and solve complex problems, it also presents significant challenges, particularly in the realm of fairness and equity. A growing concern is the presence of bias in AI algorithms, which can perpetuate existing inequalities and amplify harmful stereotypes.
Algorithmic bias, also known as machine learning bias or AI bias, refers to the systematic and unfair discrimination exhibited by AI systems against certain individuals or groups. This bias arises from the inherent limitations and biases present in the data used to train AI models, as well as from the algorithms themselves. The consequences of algorithmic bias can be profound, ranging from discriminatory hiring practices to biased loan approvals, and even impacting the administration of justice.
This paper delves into the multifaceted issue of bias in AI, exploring its origins, impacts, and potential solutions. We will examine the various sources of bias, including data bias, algorithmic bias, and user bias, and analyze the consequences of such bias on individuals, communities, and society as a whole. We will then discuss strategies for mitigating algorithmic bias and explore ethical and legal considerations surrounding its use. Finally, we will look towards the future of AI and fairness, considering how we can ensure that AI systems are developed and deployed in a responsible and equitable manner.
Sources of Bias in AI
Algorithmic bias stems from various sources, each contributing to the systematic unfairness exhibited by AI systems. Understanding these sources is crucial for developing strategies to mitigate bias and ensure fairness in AI applications.
- Data Bias: The most common source of bias in AI arises from the data used to train algorithms. If the training data reflects existing societal biases, the resulting AI model will inevitably inherit and amplify these biases. For instance, if a hiring algorithm is trained on data from a company with a predominantly male workforce, it may learn to favor male candidates, perpetuating gender inequality.
- Algorithmic Bias: Even with unbiased data, the algorithms themselves can introduce bias. This can occur due to the design choices made by developers, the inherent limitations of certain algorithms, or the way algorithms are applied to specific tasks. For example, a facial recognition algorithm trained on a dataset primarily composed of light-skinned individuals may struggle to accurately identify darker-skinned individuals, leading to discriminatory outcomes.
- User Bias: Human users can also introduce bias into AI systems through their interactions with the technology. For example, a search engine algorithm might be biased towards certain search terms based on the preferences and biases of its users. This can lead to the reinforcement of existing stereotypes and prejudices.
- Sampling Bias: The selection of data used to train AI models can introduce bias if the sample is not representative of the population it intends to serve. For instance, a medical AI model trained on data from a predominantly white population may not perform as well on individuals from other racial or ethnic backgrounds.
- Temporal Bias: Data collected over time can reflect historical biases and inequalities. If an AI model is trained on data that captures historical discrimination, it may perpetuate these biases in its predictions. For example, a loan approval algorithm trained on historical data may continue to discriminate against certain groups, even if current lending practices are more equitable.
The interplay of these sources of bias creates a complex and multifaceted problem. Recognizing and addressing each source is essential for developing AI systems that are fair, equitable, and beneficial for all.
Impact of Algorithmic Bias
The consequences of algorithmic bias are far-reaching and can have a profound impact on individuals, communities, and society as a whole. These biases can lead to discriminatory outcomes, exacerbating existing inequalities and hindering progress toward a more just and equitable world. The impact of algorithmic bias can be observed in various domains, including:
- Hiring and Employment: AI-powered hiring tools are increasingly used to screen candidates, but if these tools are biased, they can perpetuate discrimination against certain groups, leading to unfair hiring decisions and limiting opportunities for underrepresented individuals. This can contribute to the perpetuation of workforce inequalities based on race, gender, or other protected characteristics.
- Criminal Justice: Algorithmic bias in criminal justice systems can lead to unfair sentencing, discriminatory policing practices, and biased risk assessments. For example, algorithms used to predict recidivism rates may disproportionately target individuals from marginalized communities, leading to unfair incarceration and surveillance practices.
- Financial Services: AI algorithms are used in financial decision-making, such as loan approvals, credit scoring, and investment strategies. If these algorithms are biased, they can result in unfair access to credit, discriminatory lending practices, and unequal investment opportunities, further entrenching socioeconomic disparities.
- Healthcare: AI in healthcare holds great promise, but biased algorithms can lead to misdiagnosis, unequal access to care, and disparities in treatment outcomes. For example, an algorithm used to predict disease risk may be less accurate for individuals from certain racial or ethnic groups, leading to delayed or inadequate care.
- Education: AI algorithms are used to personalize learning experiences, but biased algorithms can lead to unequal educational opportunities. For example, algorithms used to assign students to different tracks or programs may be biased against students from disadvantaged backgrounds, limiting their access to advanced courses and opportunities.
It’s crucial to recognize that the impact of algorithmic bias goes beyond individual cases. It has the potential to perpetuate and amplify existing social inequalities, hindering progress toward a fairer and more just society.
Mitigating Algorithmic Bias
Addressing algorithmic bias requires a multifaceted approach that involves interventions at various stages of the AI development and deployment lifecycle. Mitigating bias is not about eliminating differences in outcomes, but rather ensuring that those differences are not the result of unfair or discriminatory treatment. Strategies for mitigating algorithmic bias include:
- Data Quality and Fairness: Ensuring the quality and fairness of training data is paramount. This involves identifying and mitigating biases in the data collection process, such as ensuring representation of diverse groups and removing irrelevant or discriminatory features. Techniques like data augmentation, re-weighting, and adversarial learning can be employed to address data imbalances and mitigate bias in the training data.
- Algorithm Design and Selection: Choosing algorithms that are less susceptible to bias and incorporating fairness constraints into the algorithm design can help prevent bias from being introduced during the development process. Techniques like fair ranking, calibrated classification, and counterfactual fairness can be applied to ensure that algorithms make fair decisions.
- Transparency and Explainability: Transparency and explainability in AI models are crucial for identifying and addressing bias. Providing insights into how an algorithm makes decisions allows for the detection of potential biases and enables stakeholders to understand the underlying logic behind the model’s predictions. Techniques like model interpretability, feature attribution, and decision rules can help explain the model’s behavior and expose potential biases.
- Auditing and Monitoring: Regularly auditing and monitoring AI systems for bias is essential to detect and address any emerging issues. This involves testing the algorithm on diverse datasets, assessing its performance across different demographic groups, and identifying any systematic disparities in outcomes. Continuous monitoring allows for the identification and correction of bias over time.
- Human Oversight and Collaboration: While AI can play a crucial role in decision-making, human oversight and collaboration are essential for mitigating bias. Human experts can review the results of AI systems, provide feedback, and ensure that the technology is used ethically and responsibly. Involving diverse perspectives in the development and deployment of AI systems can help identify and address biases that might otherwise go unnoticed.
Mitigating algorithmic bias is an ongoing process that requires continuous attention and effort. By implementing these strategies, we can work towards creating AI systems that are fair, equitable, and beneficial for all.
Ethical and Legal Considerations
The development and deployment of AI systems raise significant ethical and legal considerations, particularly in the context of algorithmic bias. Addressing these considerations is crucial for ensuring that AI is used responsibly and ethically, promoting fairness and minimizing harm. Key ethical and legal considerations include:
- Accountability and Transparency: Who is accountable for the consequences of biased AI systems? It is essential to establish clear lines of accountability for the development, deployment, and use of AI, including identifying the responsible parties for any discriminatory outcomes. Transparency in AI algorithms and data usage is also crucial, allowing for the detection of bias and the understanding of how decisions are made.
- Fairness and Non-discrimination: AI systems should be designed and deployed in a way that promotes fairness and non-discrimination. This involves ensuring that algorithms do not perpetuate existing biases or create new forms of discrimination. Legal frameworks and regulations should be developed to address algorithmic discrimination, prohibiting the use of AI systems that result in unfair or discriminatory outcomes.
- Privacy and Data Protection: The use of AI often involves the collection and analysis of personal data, raising concerns about privacy and data protection. Legal frameworks should be strengthened to protect individuals’ privacy rights, ensuring that data is collected, used, and stored in a responsible and ethical manner. Data governance principles should be established to ensure that data is used for legitimate purposes and that individuals have control over their personal information.
- Human Rights and Dignity: AI systems should respect human rights and dignity. This includes ensuring that AI is not used to exploit or harm individuals, and that it is used in a way that promotes human autonomy and well-being. Ethical frameworks should be developed to guide the use of AI in a way that upholds human rights and dignity.
- Access and Equity: AI systems should be accessible to all and should not exacerbate existing inequalities. Efforts should be made to ensure that AI benefits all members of society, regardless of their socioeconomic background, race, gender, or other protected characteristics. Policies and initiatives should be implemented to promote equitable access to AI technologies and resources.
Addressing these ethical and legal considerations is essential for ensuring that AI is developed and deployed responsibly and ethically. Collaboration between policymakers, technologists, ethicists, and legal experts is crucial to create a framework that promotes fairness, accountability, and respect for human rights in the age of AI.
Case Studies of Algorithmic Bias
Real-world examples of algorithmic bias provide compelling evidence of the potential harms of biased AI systems and highlight the need for robust mitigation strategies. These case studies demonstrate how seemingly neutral algorithms can perpetuate and amplify existing societal inequalities, leading to discriminatory outcomes.
- COMPAS (Correctional Offender Management Profiling for Alternative Sanctions): This risk assessment tool, used by the US criminal justice system to predict recidivism rates, has been shown to be biased against Black defendants. Studies have found that COMPAS assigns higher risk scores to Black defendants compared to white defendants with similar criminal histories, leading to harsher sentencing and increased incarceration rates for Black individuals.
- Facial Recognition Systems: Facial recognition algorithms have been found to be less accurate in identifying individuals with darker skin tones, particularly women and people of color. This bias can lead to discriminatory outcomes in law enforcement, border control, and other applications, where facial recognition is used for identification and surveillance purposes.
- Google’s Search Algorithm: A study by researchers at the University of Washington found that Google’s search algorithm displayed racial bias, showing more results for stereotypically “black” names (like DeShawn) when searching for terms associated with crime compared to stereotypically “white” names (like Brad). This bias can reinforce existing stereotypes and contribute to racial profiling.
- Hiring Algorithms: AI-powered hiring tools have been found to perpetuate gender bias. For example, Amazon abandoned its AI recruiting tool after it was discovered that the system was biased against female candidates, favoring male applicants. This bias stemmed from the fact that the algorithm was trained on historical hiring data, which reflected a predominantly male workforce in the tech industry.
- Loan Approval Algorithms: AI algorithms used to assess loan applications have been found to discriminate against individuals from marginalized communities. For instance, algorithms that use factors like credit score and income may be biased against individuals with lower credit scores or incomes, who are disproportionately from minority groups. This can lead to unequal access to credit and financial opportunities.
These case studies demonstrate the real-world consequences of algorithmic bias, highlighting the need for ongoing vigilance and proactive mitigation strategies. It is crucial to learn from these examples and implement rigorous measures to prevent bias from being introduced into AI systems.