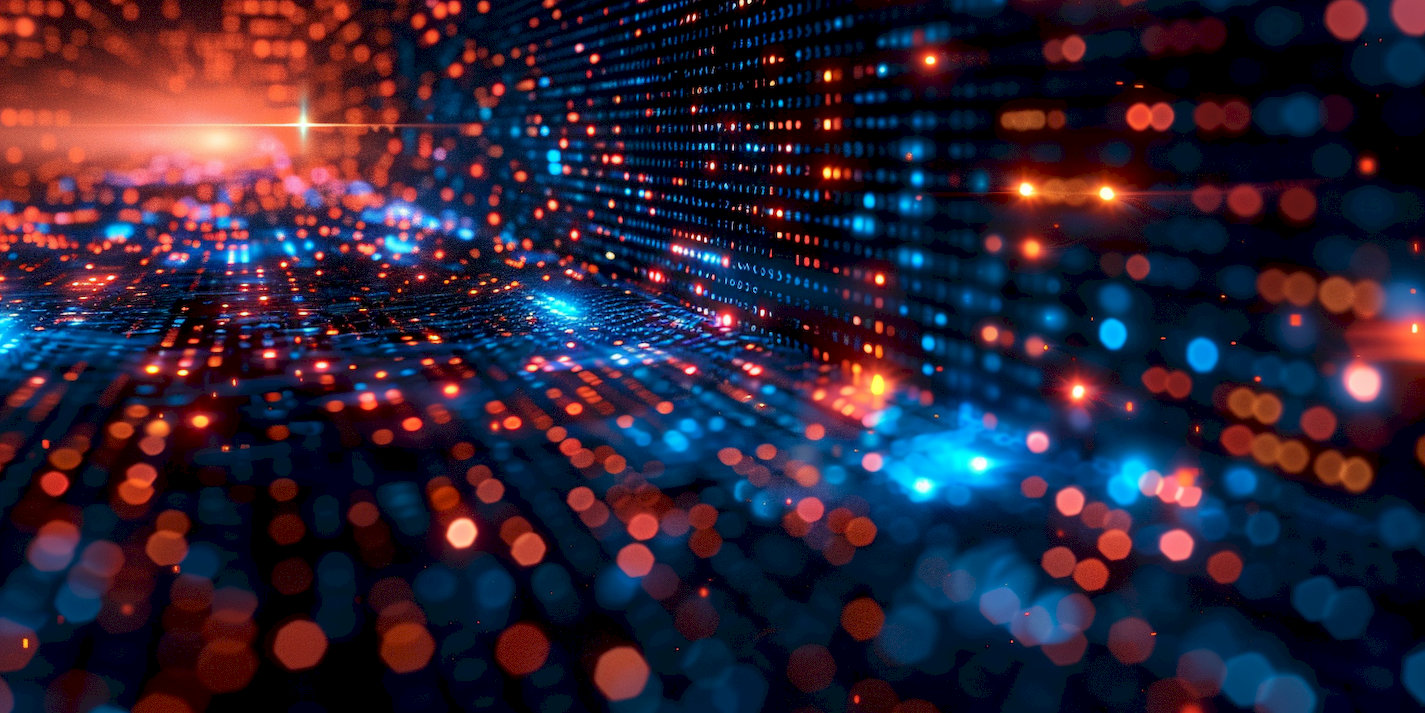
The Evolution of Machine Learning Algorithms
The field of machine learning has undergone a remarkable evolution, with innovative algorithms constantly pushing the boundaries of what is possible. Early machine learning algorithms, such as the nearest neighbor algorithm, were developed in the 1960s and used for pattern recognition tasks. These algorithms laid the foundation for the more sophisticated algorithms that followed. The development of the perceptron algorithm in 1957, an early type of artificial neuron, marked a significant step forward. The perceptron algorithm was capable of learning from data and making predictions. The development of the perceptron algorithm, an early type of artificial neuron, marked a significant step forward. The perceptron algorithm was capable of learning from data and making predictions.
Deep Learning: A Powerful Subset of Machine Learning
Deep learning, a powerful subset of machine learning, has emerged as a transformative force in recent years, driving advancements across various domains. Deep learning algorithms, inspired by the structure and function of the human brain, leverage artificial neural networks with multiple layers to learn complex patterns and representations from data. This ability to extract intricate features from raw data, such as images, text, and audio, has led to remarkable breakthroughs in tasks like image recognition, natural language processing, and speech synthesis.
The “deep” in deep learning refers to the use of multiple layers in the network, enabling the extraction of increasingly abstract features as the data flows through the layers. These architectures, including deep neural networks, deep belief networks, recurrent neural networks, and convolutional neural networks, have revolutionized machine learning by enabling the development of highly accurate and robust models. Deep learning has proven particularly effective in tasks involving unstructured data, where traditional machine learning methods often struggle. This has led to its widespread adoption in various fields, including computer vision, natural language processing, robotics, and healthcare.
The success of deep learning is attributed to several factors, including the availability of large datasets, advancements in computing power, and the development of new algorithms and optimization techniques. The ability of deep learning models to learn from massive amounts of data, coupled with the increasing availability of computational resources, has enabled the training of increasingly complex and sophisticated models. Deep learning continues to evolve rapidly, with researchers exploring new architectures, optimization methods, and applications. The potential of deep learning to solve complex problems and drive innovation across various fields is immense, making it a key area of research and development in the field of artificial intelligence.
The Impact of AI Algorithms on Machine Learning
The advent of AI algorithms has profoundly impacted the landscape of machine learning, ushering in a new era of possibilities. AI algorithms, particularly those rooted in deep learning, have revolutionized the way machines learn and solve complex problems. These algorithms have empowered machine learning models to achieve unprecedented levels of accuracy and efficiency in diverse applications, from image recognition and natural language processing to medical diagnosis and scientific discovery.
The impact of AI algorithms on machine learning can be observed in several key areas. First, AI algorithms have significantly enhanced the ability of machine learning models to handle complex and high-dimensional data. Deep learning architectures, with their ability to learn intricate representations from vast amounts of data, have enabled the development of models that can handle data complexities that were previously intractable. Second, AI algorithms have led to the development of more efficient and scalable machine learning models. The advancements in optimization techniques and computational resources have facilitated the training of models on massive datasets, enabling the creation of models that can be deployed at scale.
Furthermore, AI algorithms have fostered the development of new machine learning techniques, such as generative AI, which allows for the creation of novel data instances. Generative AI models, trained on large datasets, can generate realistic images, text, and audio, opening up new possibilities in areas like content creation, design, and scientific exploration. AI algorithms have also spurred the development of explainable AI (XAI) techniques, which aim to make the decision-making processes of machine learning models more transparent and understandable. XAI is crucial for building trust and ensuring responsible use of AI in sensitive applications such as healthcare and finance.
Revolutionizing Scientific Discovery with AI
The integration of AI algorithms into scientific discovery is transforming the way research is conducted, accelerating breakthroughs and unlocking new frontiers of knowledge. AI is empowering scientists to tackle complex problems, analyze vast datasets, and generate novel insights that were previously unattainable through traditional methods. The application of AI in scientific research is not limited to a single discipline but spans across various fields, from drug discovery and materials science to astrophysics and climate modeling.
One of the most significant impacts of AI on scientific discovery is the ability to analyze massive datasets, often referred to as “big data.” AI algorithms, particularly those based on machine learning and deep learning, can efficiently process and extract meaningful patterns from vast amounts of data, revealing hidden correlations and generating hypotheses that might not be apparent to human researchers. This capability is particularly valuable in fields like genomics, where analyzing massive datasets of genetic information can lead to the identification of disease-related genes and the development of personalized medicine.
Another transformative aspect of AI in scientific discovery is its ability to automate tasks and accelerate research processes. AI algorithms can be used to design experiments, analyze results, and even generate new hypotheses, freeing up scientists to focus on higher-level tasks such as interpretation and knowledge synthesis. AI-powered tools are also revolutionizing scientific visualization, enabling researchers to explore complex data and gain deeper insights through interactive visualizations and simulations. As AI continues to evolve, its potential to revolutionize scientific discovery will only continue to grow, promising a future where AI and human ingenuity collaborate to unlock the mysteries of the universe.
Generative AI: A New Era of Machine Learning
Generative AI, a burgeoning field within machine learning, has emerged as a powerful force, enabling the creation of novel data instances that mimic real-world examples. These AI models, trained on vast datasets, can generate realistic images, text, audio, and even code, opening up a new era of possibilities in various domains. Generative AI algorithms leverage deep learning architectures, particularly those based on generative adversarial networks (GANs) and transformers, to learn intricate patterns and representations from data, allowing them to generate highly realistic and coherent outputs.
The impact of generative AI is far-reaching, extending across diverse industries and research areas. In the creative arts, generative AI is being used to generate paintings, music, and literature, pushing the boundaries of artistic expression and inspiring new forms of creativity. In design and engineering, generative AI is being used to design innovative products, optimize manufacturing processes, and explore new materials. Generative AI is also revolutionizing scientific discovery, enabling researchers to generate new drug candidates, design advanced materials, and simulate complex physical phenomena.
However, the rise of generative AI also presents ethical challenges, raising concerns about the potential for misuse and the impact on creativity and authenticity. It is crucial to ensure that generative AI is developed and deployed responsibly, with safeguards in place to mitigate potential risks and promote ethical use. As generative AI continues to evolve, it will undoubtedly play an increasingly significant role in shaping the future, impacting industries, research, and even the way we perceive and interact with the world around us.
Applications of AI Algorithms in Education
The application of AI algorithms in education is transforming the learning landscape, offering personalized and engaging experiences for students of all ages. AI-powered tools are revolutionizing various aspects of education, from personalized learning and adaptive assessments to automated grading and student support. AI algorithms, particularly those based on machine learning and natural language processing, are playing a crucial role in creating intelligent systems that can adapt to individual student needs and provide tailored learning pathways.
Personalized learning, powered by AI, tailors educational content and pace to each student’s unique learning style, strengths, and weaknesses. AI algorithms analyze student data, such as performance on assessments, engagement patterns, and learning preferences, to identify individual needs and provide personalized recommendations. Adaptive assessments, another application of AI in education, adjust the difficulty level of assessments in real-time based on student performance, providing a more accurate and effective measure of learning. AI-powered chatbots and virtual assistants are also being deployed to provide students with 24/7 support, answering questions, offering guidance, and providing personalized feedback.
AI algorithms are also being used to automate grading and provide feedback on student work, freeing up teachers to focus on more engaging and personalized interactions with students. Furthermore, AI-powered tools are being used to detect plagiarism, identify students at risk of academic difficulties, and even predict student success in future courses. While the potential of AI in education is immense, it is crucial to address ethical concerns and ensure that AI is used in a way that benefits all students and promotes equitable learning opportunities.
The Future of AI Algorithms in Machine Learning
The future of AI algorithms in machine learning is brimming with exciting possibilities, promising a world where machines learn and solve problems with ever-increasing sophistication and efficiency. As research and development in AI continue to advance at a rapid pace, we can anticipate significant breakthroughs in areas such as explainable AI, federated learning, and reinforcement learning, shaping the future of machine learning and its impact on various domains.
Explainable AI (XAI) is poised to play a crucial role in addressing the “black box” problem, making AI models more transparent and understandable. XAI techniques aim to provide insights into the decision-making processes of AI models, enabling users to understand why a model makes a particular prediction or recommendation. This is particularly important for building trust in AI systems, especially in sensitive applications such as healthcare and finance. Federated learning, another promising area of research, allows multiple devices or systems to collaboratively train AI models without sharing their raw data. This approach is particularly valuable for protecting privacy and data security, especially in healthcare settings where sharing sensitive patient data is often restricted.
Reinforcement learning, which enables machines to learn through trial and error, is also expected to play a more significant role in the future of machine learning. Reinforcement learning algorithms are being used to train AI agents to perform complex tasks, such as playing games, controlling robots, and optimizing complex systems. As AI algorithms become more sophisticated and capable, we can expect to see them deployed in increasingly complex and challenging applications, transforming industries, driving innovation, and shaping the future of human society.
The Transformative Power of AI Algorithms
The transformative power of AI algorithms is undeniable, revolutionizing machine learning and shaping the future across various domains. From deep learning architectures that enable machines to learn intricate patterns from complex data to generative AI models that create novel content, innovative AI algorithms are driving unprecedented progress in areas such as image recognition, natural language processing, scientific discovery, and even artistic expression. The impact of AI is not limited to specific fields but is reshaping the way we live, work, and interact with the world around us.
While the potential of AI algorithms is immense, it is crucial to approach their development and deployment with a sense of responsibility and ethical awareness. Addressing concerns about bias, privacy, and potential misuse is paramount to ensure that AI benefits all of humanity. As AI continues to evolve, it is essential to foster collaboration among researchers, policymakers, and industry leaders to ensure that AI is developed and used in a way that promotes ethical, responsible, and equitable outcomes. The future of AI is bright, and by harnessing its transformative power responsibly, we can unlock a future where AI empowers humanity to solve complex challenges, drive innovation, and create a better world for all.